Topic:Cross-Domain Learning and Information Recommendation
Lecturer:Prof. Xingquan Zhu, UTS
Time:2011.3.15,Tuesday, 14:30 - 16:00 p.m
Location:New Main Building A1148
Host:Associate Professor Wu Junjie
Abstract:
Due to the increasing ability for data collection and the increasing complexity of real-world problems, applications are facing the challenge of sharing information across multiple domains and multiple sources to achieve a common goal. Examples include collaborative filtering where rating matrices collect information from different user groups on items from different domains, and in semi-supervised learning where unlabeled samples are collected from domains different from the labeled instances. In this talk, I will address cross-domain learning from both collaborative filtering and semi-supervised learning perspectives. For collaborative filtering, we regard user rating matrices are generated from some hidden models, and our goal is to recover generative models hidden behind the rating matrices across different domains. For semi-supervised learning, we regard that both labeled and unlabeled samples are generated from some hidden concepts with labeling information partially observable for some samples. Our objective is to recover the hidden concepts, and take them as new features to link labeled and unlabeled samples for learning. Because unlabeled samples are only used to generate new features, but not to be explicitly included in the training set, our method overcomes the inherent disadvantages of the traditional methods, especially for samples not within the same domain as the labeled instances. Experimental results and comparisons demonstrate the performance of the proposed approach for cross-domain information sharing and recommendation.
Lecturer:
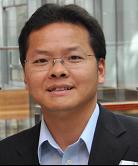
Xingquan Zhu, Ph.D, Professor
University of Technology, Sydney
xqzhu@it.uts.edu.au|
Xingquan Zhu is a Professor at the Centre for Quantum Computation and Intelligent Systems, University of Technology, Sydney. He is the recipient the Australia ARC Future Fellowship (Level 2). He received his B.S. and M.S. degrees in Communication Engineering from Xidian University, and his PhD degree in Computer Science from Fudan University, Shanghai China, in 2001. He was a Postdoctoral Associate with the Department of Computer Science, Purdue University, West Lafayette, USA, from 2001 to 2002, a Research Assistant Professor with the Department of Computer Science, University of Vermont, USA, from 2002 to 2006, a tenure track Assistant Professor with the Department of Computer Science and Engineering, Florida Atlantic University, USA, from 2006 to 2009, and an Associate Professor at the Faculty of Engineering and Information Technology, University of Technology, Sydney, from 2009 to 2010. Since 2000, he has published more than 110 referred journal and conference proceeding papers. His research mainly focuses on data mining, machine learning, multimedia systems, and bioinformatics.
Dr. Zhu has been an Associate Editor of the IEEE Transactions on Knowledge and Data Engineering since 2009 and is a Program Committee Co-chairs for the 23rd IEEE International Conference on Tools with Artificial Intelligence (ICTAI 2011), the 9th International Conference on Machine Learning and Applications (ICMLA 2010). He also serves (or served) as program vice chairs, finance chairs, publicity co-chairs, program committee members for many international conferences, including KDD, ICDM, and CKIM.
School of Economics & Management
2011-03-09