Title:Estimating the Helpfulness of Online Product Reviews: An Iterative Bayesian Probability Approach
Presenter:Xunhua Guo
Host:Professor Hao Jinxing
Time:2019.4.10 12:15-13:30
Location:A618
Abstract:
Voting mechanisms are widely adopted for evaluating the quality and reputation of user generated content, such as online product reviews. For the reviews that do not receive sufficient votes, techniques and models are developed to automatically assess their helpfulness levels. Existing methods are mostly centered on feature analysis, ignoring the information conveyed in the frequencies and patterns of user votes. Consequently, the accuracy of helpfulness measurement is limited. Inspired by related findings from prediction theories and consumer behavior research, we propose a novel approach characterized by the technique of iterative Bayesian distribution estimation, aiming to more accurately measure the helpfulness levels of reviews used for training prediction models. Using synthetic data and a real-world data set involving 1.67 million reviews and 5.18 million votes from Amazon, a simulation experiment and a two-stage data experiment show that the proposed approach outperforms existing methods on accuracy measures. An out-of-sample user study is conducted on Amazon Mechanical Turk as well as in a university lab. The results further illustrate the predictive power of the new approach. Practically, the research contributes to e-commerce by providing an enhanced method for exploiting the value of user-generated content. Academically, we contribute to the design science literature with the novel approach that may be adapted to a wide range of research topics such as recommender systems and social media analytics.
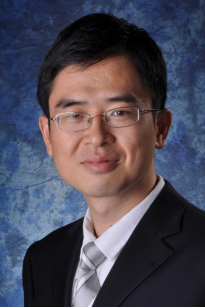