Prof.Zhengjun Zhang:Simulated Distribution Based Learning for Non-regular and Regular Statistical Inferences
On 21st May, 2019, we welcomeProf.ZhengjunZhangto our series lecture on “Economics and Business”, as well as his speechon Simulated Distribution Based Learning for Non-regular and Regular Statistical Inferences.Zhengjun Zhang is Professor at Department of Statistics in the University of Wisconsin-Madison, Associate Editor for the ournal of Business &Economic Statisticsand Associate Editor for theStatistica Sinica. He obtained his Ph.D both from University of North Carolina at Chapel Hill and from Beihang University in Management Science and Engineering. He is interested inresearch on financial time series analysis, extreme value theory, abnormal climate analysis,rare disease analysis, financial risk modeling and evaluation, market systemic risk assessment and so on. The lecture was hosted by Associate Dean Haitao Zheng from School of Economics and Management at Beihang University.
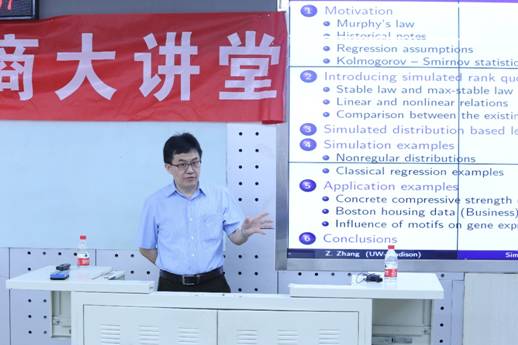
In the following lecture,Prof. Zhengjun Zhangestablishes a fundamental theorem which guarantees the transformed order statistics (to a given marginal) from the assumed distribution of a random variable (or an errorterm) to be arbitrarily close to the order statistics of a simulated sequence of the same marginal distribution. Different from the Kolmogorov-Smirnov test which is based on absolute errors between the empirical distribution and the assumed distribution, the statistics proposed in the paper are based on relative errors of the transformed order statistics to the simulated ones. Uponusing the constructed statistic (or the pivotal quantity in estimation) as a measure of the relative distance between two ordered samples, we estimate parameters such that the distance is minimized. Unlike many existing methods,e.g., maximum likelihood estimation,which rely on some regularity conditionsand/or the explicit form of probability density function, the new method only assumes a mild condition that the cumulative distribution function can be approximated to a satisfiedprecision. The paper illustrates simulation examples to show its superior performance. Under the linear regression settings, the proposed estimation performs exceptionallywell regarding preserving the error terms (i.e., the residuals) to be normally distributed which is a fundamental assumption in the linear regression theory andapplications.
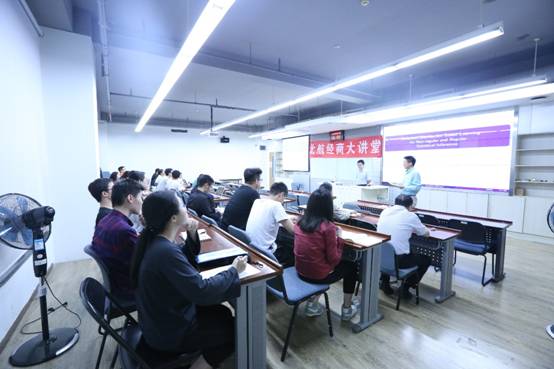
During and after the lecture, more than 30 scholars and students from BUAA SEM discussed the key points of the talk with Prof. Zhengjun Zhang, and also raised quite a few relevant questions,which provoke further thinking on the issue from both parties.